First Things First
Targets and Traps
With the rapid decline of global biodiversity and the increasing threat of species extinction, there is a growing interest in accurately and efficiently monitoring the health and distribution of species and ecosystems. Traditional field surveys involve audio-visual surveys or trapping in the field to document the presence, abundance and distribution of species. These methods provide high quality information, but they are labour intensive and therefore limited in area and time covered. Alternatively, there’s remote sensing1. Remote sensing technologies such as satellite imagery, LiDAR and drones can provide valuable data on habitat characteristics, land cover changes and ecosystem health. They can cover large areas, but provide less specific information.
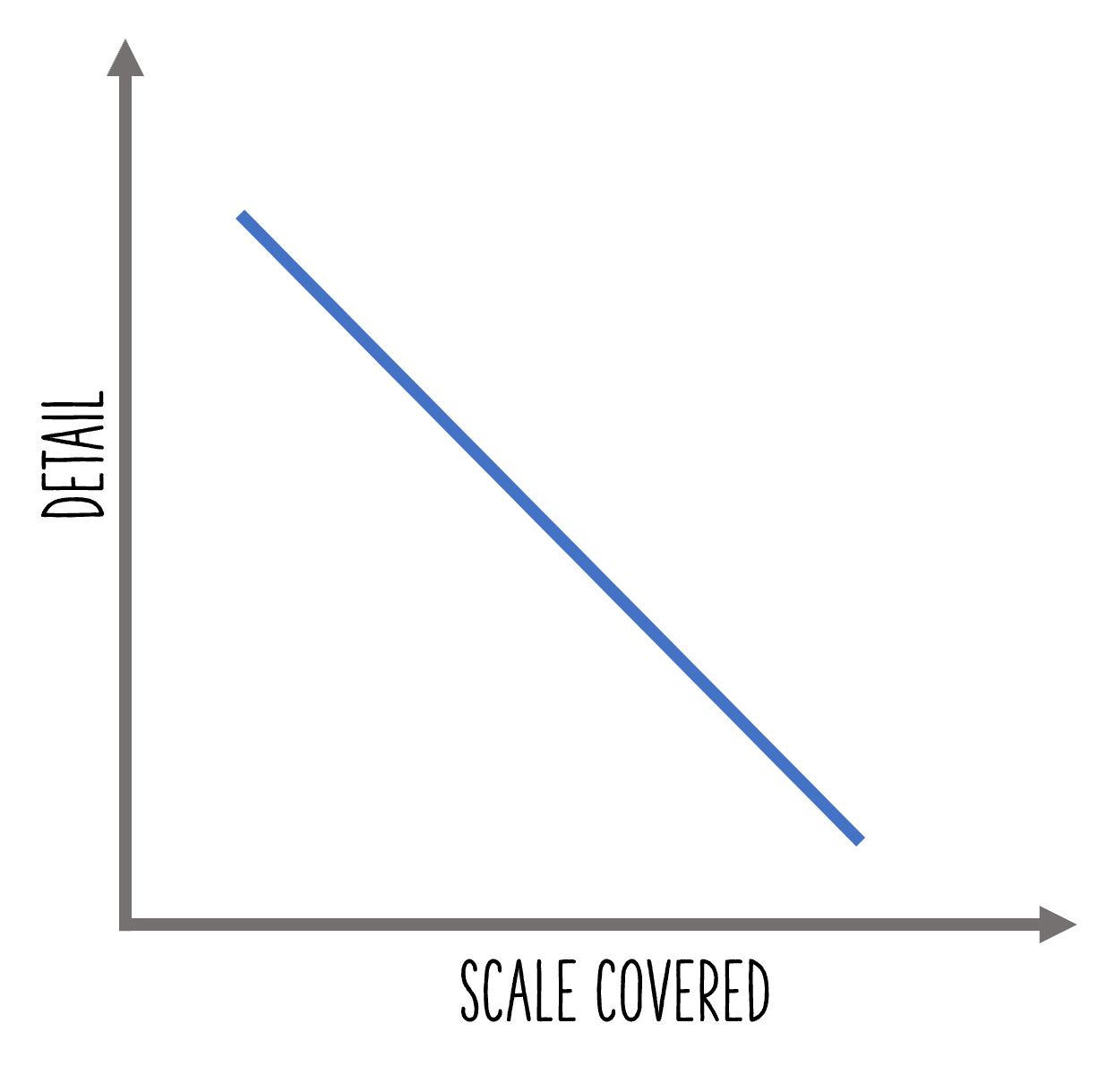
In the middle is what we’ll call proximate sensing of biodiversity. In proximate sensing, compared to remote sensing, the sensor is close to the object and is installed on platforms ranging from handheld, fixed installations or robotics to tractor-embedded sensors. The (semi-)automated collection of data from the field, often using cameras, microphones or other sensors, allows information to be gathered on species populations, biodiversity, phenological shifts and interactions. In theory, this non-invasive approach should allow scientists to observe and analyze their target with minimal effort2. However, there are many challenges to developing a fully functional, autonomous, proximate sensor pipeline.
In this introductory course, we will explore the principles and methodologies of proximate sensing in biodiversity research, highlighting its potential benefits and limitations. We will also discuss various sensing techniques commonly employed in biodiversity studies, along with case studies demonstrating their practical applications in conservation.
The construction of a device for the proximate sensing of biodiversity requires expertise from a number of disciplines for the successful design, development, deployment and maintenance of the system. Understanding ecological principles and biodiversity metrics is crucial for designing monitoring protocols, selecting appropriate sensors, and interpreting monitoring data accurately. Expertise in sensor technology is essential for selecting, deploying, and maintaining sensors. This includes knowledge of sensor types, data acquisition techniques, and sensor network design. Pure engineering skills are needed to build a lasting senor box. Software engineering skills are necessary for developing the software infrastructure and applications that support automated biodiversity monitoring systems. Lastly, data science expertise is required to process, analyze, and interpret the large volumes of data generated by biodiversity sensors.
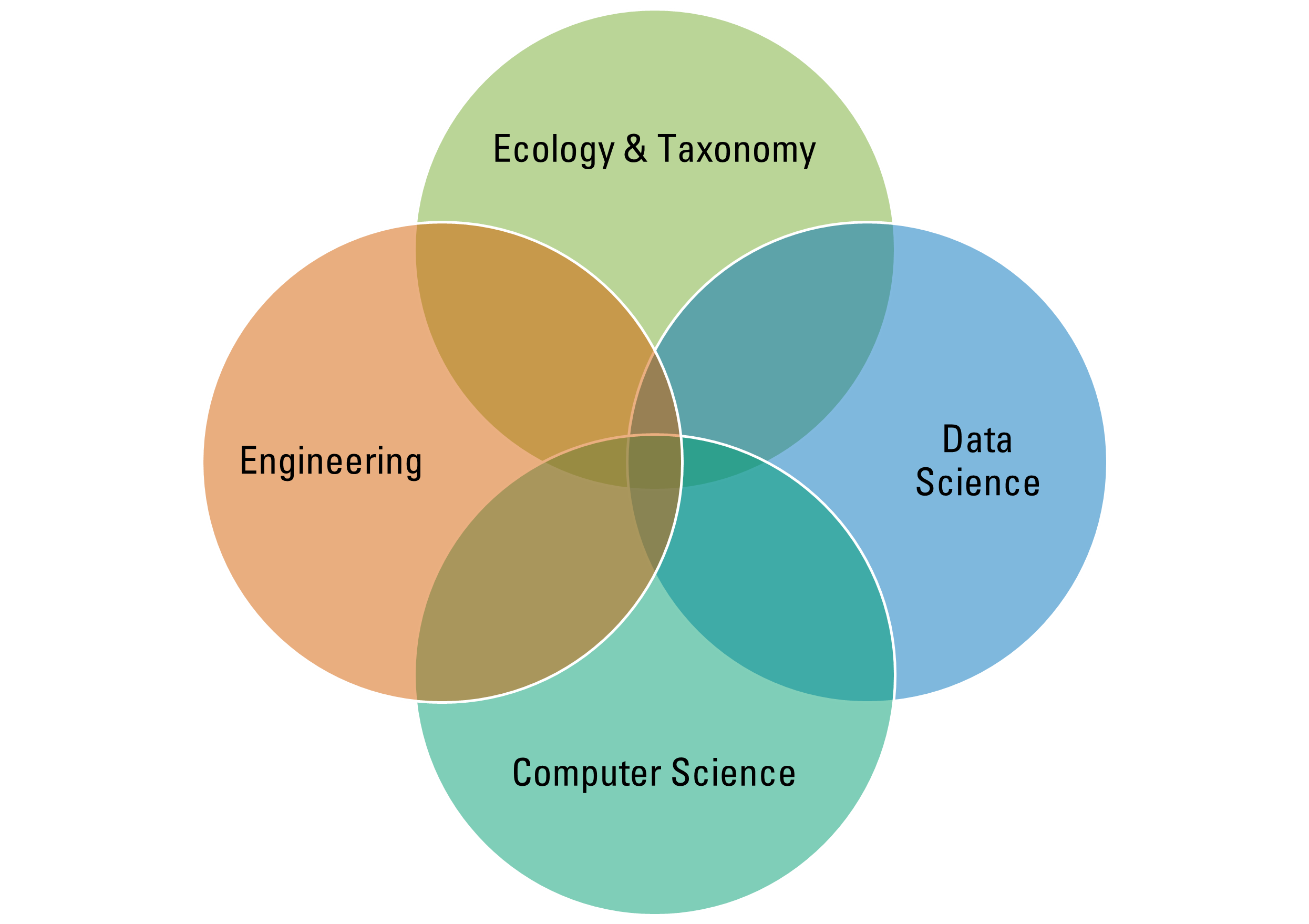
Please note that this course does not have the specialist staff to cover each of these aspects in detail. The focus lies on being able to evaluate the rapid development in this area with an alert mind and to encourage students to assess the sustainable development and utilization of these new technologies.
This first unit will give you some general background, i.e. what proximate sensing of biodiversity is, how it compares to traditional monitoring, and how the target animal can affect the type of sensor box used.
Learning objectives
At the end of this unit you should be able to:
- Explain the concept of proximate sensing and its significance in biodiversity conservation.
- Compare and contrast automated monitoring with traditional monitoring techniques, highlighting advantages and limitations of each approach.
- Propose sensor deployment strategies based on understanding of target species behaviors and habitat preferences.
A very good start to the literature can be found here.
Comments?
You can leave comments below if you have questions or remarks about any of the text or code in this unit. Please copy the corresponding line into your comment to make it easier to answer your question.